Radosavovic I, Dollár P, Girshick R, et al. Data distillation: Towards omni-supervised learning[C]//Proceedings of the IEEE Conference on Computer Vision and Pattern Recognition. 2018: 4119-4128.
1. Overview
1.1. Motivation
- Semi-supervised simulates labeled/unlabeled data (upper-bounded on full annotated data)
- Omni-supervised exploits extra unlabeled data (lower-bounded on full annotated data)
- Model distillation distills knowledge from the prediction of multiple model

In this paper
- it proposed data distillation which ensembles prediction from multiple transformations of unlabeled data, using single model
- Do experiments on keypoint detection and object detection
1.2. Knowledge Distillation
- Train teacher model on large amount of labeled data (A)
- Generate annotation on unlabeled data (B) based on teacher model
- Retrain student model on data (A+B)
- Problem. training model on its own prediction can not provide meaningful information
- Solution. ensembling the prediction of the different transformation of unlabeled data (flipping, scaling)
1.3. Related Work
- Ensemble multiple model, Model Compression
- FitNet
- Cross modal distillation
- Multi-view geometry
Auto-encoder (multiple capsule)
Self-training can be used for training object detection
- Multiple views or perturbations of the data can provide useful signal for semi-supervised learning
The method of this paper are also based on multiple geometric ransformations.
2. Data Distillation
Step
- Train teacher model on labeled data (A)
- Apply teacher model to multiple transformation of unlabeled data (B)
- Ensemble the multiple prediction to get annotation
- Retrain student model on data (A+B)
2.1. Multi-transform
- multi-crop
- multi-scale
In the experiment of this paper, it used multi-scale and flipping.
2.2. Ensemble
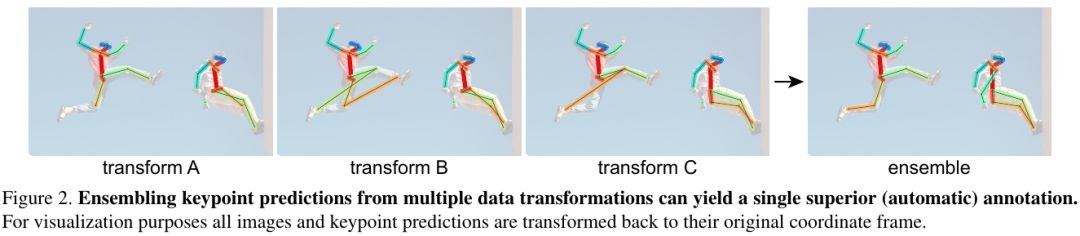
- Aggregated prediction generate new knowledge
- Aggregated prediction outperform any single prediction
2.3. Ensemble way
2.3.1. Soft Label
- average class probability
- generate probability vector, not category label
- not suitablefor structure output space (pose, detection)
2.3.2. Hard Label
- need task specific logic (NMS for merging multiple box)
3. Detail of Pose Estimation
3.1. Selecting Prediction
Generate annotation only from the prediction that are above a certain score threshold. And found that
- the average number of annotated instances per unlabeled image equal to labeled image’s (similar distribution)
- still work robust and well when not equal
3.2. Retraining
- retraining is better than fine-tuning (which is in a poor optimum).
4. Experiments
4.1. Data split
- co-80. labeled
- co-35. labeled
- co-115. co-80 + co-35
- un-120. unlabeled
- s1m-180 (sports-1M static frame). dissimilar distribution
4.2. Amount of Annotated Data
- 1:ρ in minibach.
4.3. Accuracy of Teacher Model
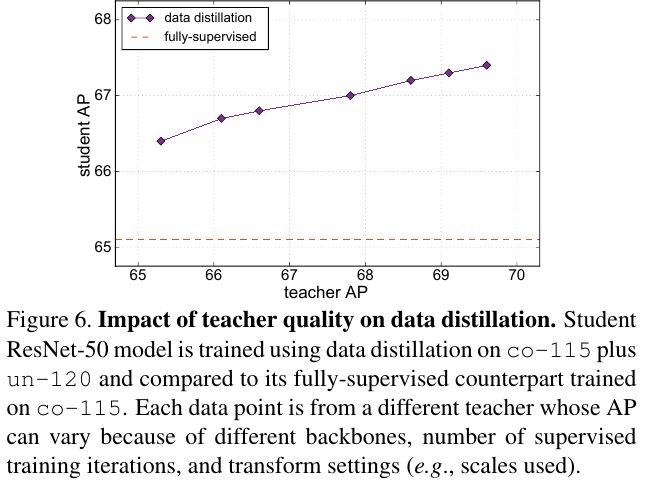
4.4. Result
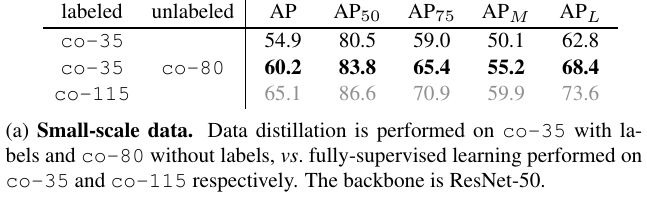

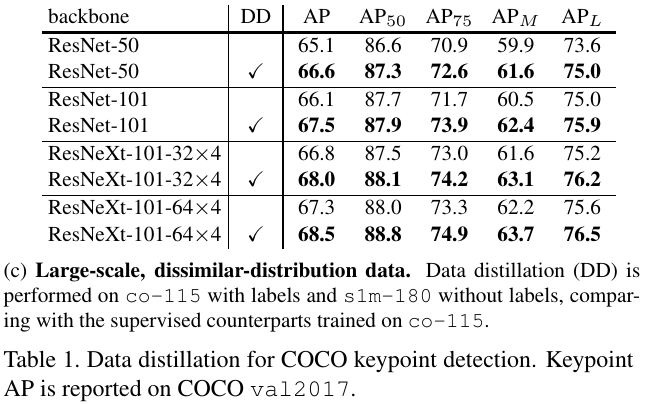
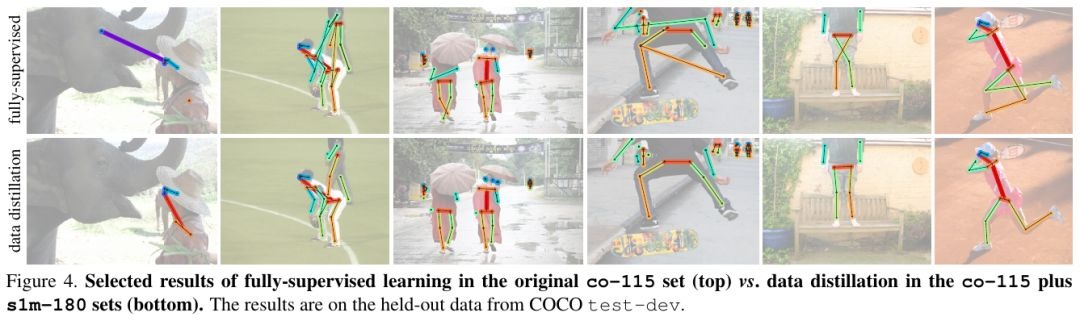